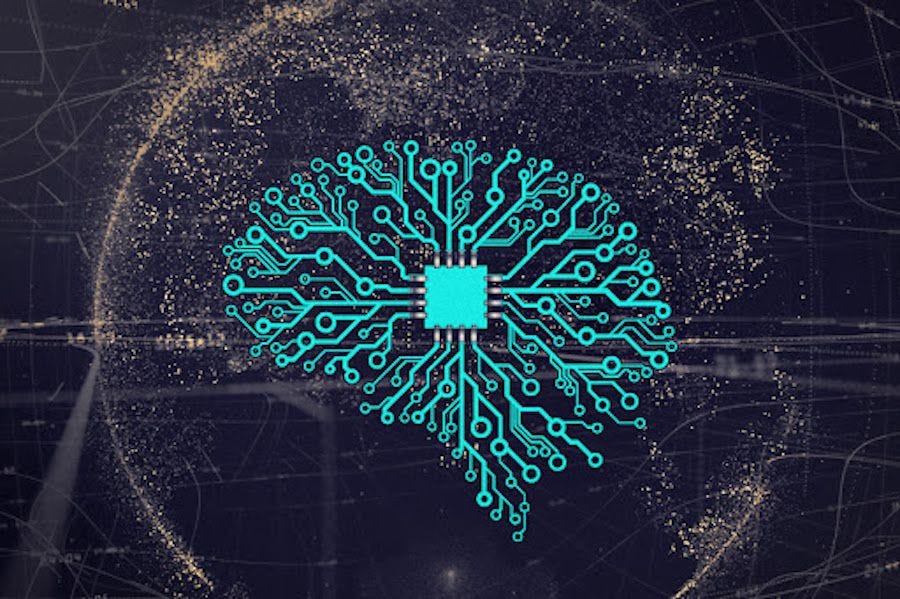
The Vatican’s official media outlet raised eyebrows Sunday when it published a report noting that synod organizers in Asia had used artificial intelligence (AI) to help draft a final document.
Vatican News was referring to the Asian synodal continental assembly in Bangkok, Thailand, held Feb. 24-26 as part of the continental stage of the global synodal process, leading to the synod on synodality in Rome this October.
The report said that Fr. Clarence Devadass, a member of the discernment and drafting team, had presented an updated draft of the final document to the assembly’s participants that was “compiled with the use of both AI and HI (Human Intelligence).”
Vatican News added that the Asia continental assembly was “the first of the continental assemblies to incorporate the use of digital technologies to gather the amendments and input from the participants.”
The report provoked debate online about the scope of AI’s role in the final document.
But in online conversations, there have been some misconceptions about the process.
Most misunderstood? The entire draft was not actually written by an AI-powered chatbot, like ChatGPT.
Instead, an AI program was used to help drafters to sift through proposals made by the assembly’s 80 participants representing 29 countries.
Did it actually help? The Pillar asked synod organizers.
‘It was effective’
Fr. Devadess, a priest based in Malaysia who has previously served as a consultor to the Dicastery for Interreligious Dialogue, said that the process began with the small groups that met throughout the assembly to discuss their responses to questions posed in the working document for the continental stage issued by the Vatican.
At the end of each discussion session, the groups were asked to submit a summary of their responses using Google Forms.
“From the data received, the responses were inputted into an AI software,” Fr. Devadess said, “and a command was given like ‘From the response below, highlight the common themes’ or ‘Which are the responses that are specific only to one particular group?’”
Fr. Nigel Barrett, a colleague of Fr. Devadess, said that another command used was “Which are the top priorities emerging from the data?”
“Once the data was sifted through, we went through the data once more, as individuals, to see if the AI-generated information was accurate,” Fr. Barrett explained.
Reflecting on the process, Fr. Devadess said: “We could safely say that it was effective in sorting the data and picking up on keywords, but it needs to be monitored by human resources to ensure the data reflected accurately the mood of the house.”
“What would have taken a couple of hours to do was done in a couple of minutes. But to ensure the integrity of the responses, it was checked again later by members of the drafting committee against the ‘raw data.’”
📰
Robots in disguise?
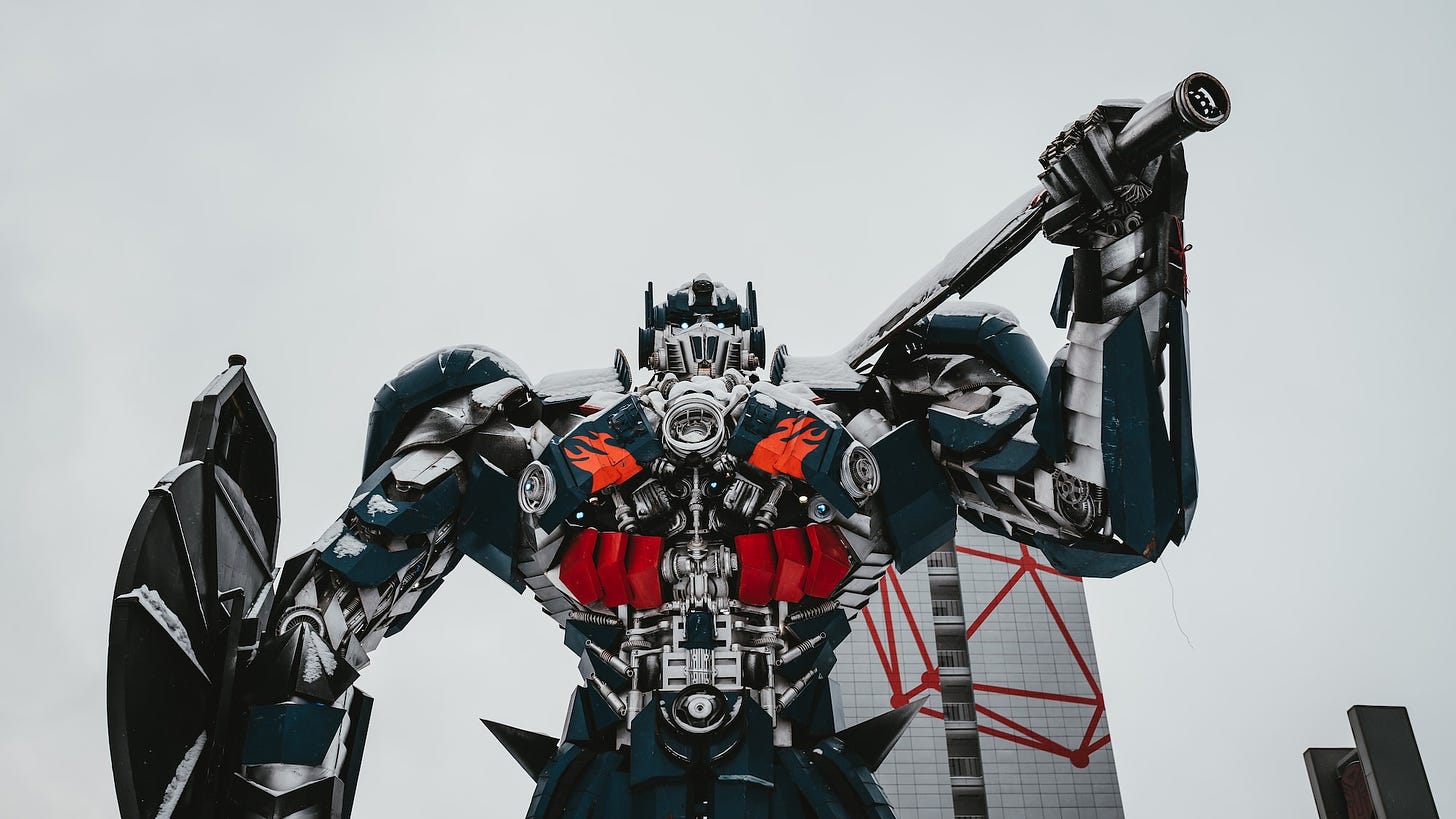
What Fr. Devadess describes appears to be an example of natural language processing, an area of artificial intelligence using machine learning to process text which has been utilized increasingly in business and academic settings.
While the phrase artificial intelligence may summon up images redolent of science fiction, the term artificial intelligence is used by data scientists to describe systems in which a computer program is designed to do tasks typically associated with humans such as identifying what a picture depicts, correctly transcribing spoken communication, or discerning meaning in written text.
Machine learning describes the subset of artificial intelligence models in which humans build an analytical model and then train it by exposing it to a large selection of data to allow it to correctly identify certain patterns, rather than directly programming the model to interpret specific signs in a designated way. This training process is always human-assisted in one way or another.
One everyday example of machine learning is the handwriting recognition software used by organizations such as the Post Office to identify addresses handwritten on letters. To train such software, a computer program is provided with millions of images depicting individual handwritten letters and numbers, and a correct identification of what character was intended in each case. Once provided with many thousands of examples of how each single character can be written, the computer identifies commonalities which it then uses to identify new, unique examples of handwritten letters and numbers. Humans continue to train the model by identifying mistakes the computer has made.
While anthropomorphic terms such as “read” or “recognize” can be misleading for what the computer does in these circumstances, the process of machine learning is in some ways modeled on human learning.
Machine learning is often driven by a type of program called a “neural network,” because it mimics human processes of recognition by storing a large number of characteristics which are taken together to identify some new piece of data.
Just as a human recognizes a written letter or number by unconsciously comparing it to all the previous examples of that letter which he or she has seen before, so the machine learning model draws on a large set of past examples to identify new ones.
The difference is that while the human has a concept of the shape itself, the computer model rapidly compares the position of individual pixels in millions of past examples to make a probabilistic estimate of how likely the new image is to belong to a particular category such as a handwritten “A”.
In natural language processing - like the kind likely used in the Asian synod meeting - the task is taking ordinary prose written by a human, and categorizing it in terms of content and even tone.
Natural language models are trained on large sets of written prose and human trainers who identify both specific topics and examples of tone. For instance, natural language models used by companies to process customer service requests are trained to identify examples of prose which are angry or sarcastic and flag those requests for additional human attention.
Super summarizing and compelling compiling?
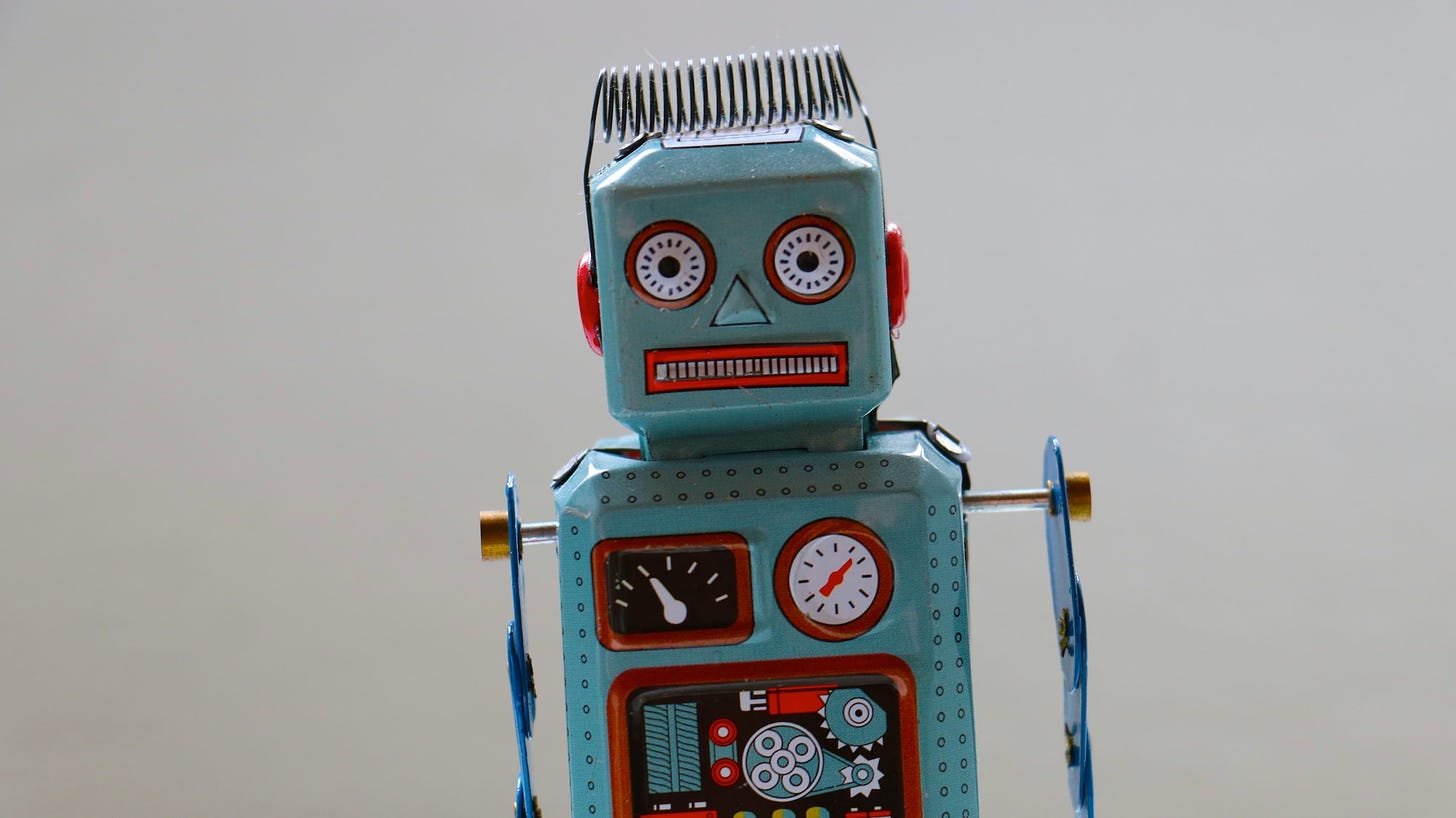
In the kind of natural language processing use by the Asian assembly, the AI model is trained to identify common topics and flag outliers. That task is similar to the technology used by The Pillar in its own analysis of the conference and global synodal documents.
As Fr. Devadess described, natural language processing is a very fast way to compile the focuses and sentiments of a large number of text submissions from different small groups.
Not only is a natural language processing model able to compile the themes and emphases of many documents much faster than a human, but the use of that kind of model removes the potential for human bias in assessing many documents.
Even without intending to, a human reader will often look for confirmation of certain hypotheses when reading a large number of documents, and the sections related to those existing focuses may stand out inordinately to the human reader, creating a bias in the summary.
It's worth noting that the kind of computer-assisted textual analysis used by synod organizers in Asia is different from the AI chatbots in the news lately, with the release of OpenAI’s ChatGPT bot and the Bing artificial intelligence tool from Microsoft which has been in widespread user testing.
AI writing tools like Bing or ChatGPT use a large training set of existing text to attempt to mimic human writing. In some sense, those models perform a summarizing function, but they are fundamentally designed to mimic human writing, not to summarize it.
But the model used by the Asian assembly was of the type designed specifically to summarize a set of documents and highlight their most common themes. That process assists the humans studying the set of texts in knowing where to look to make their own assessment.
When the Asian assembly concluded on Feb. 26, the text's final document — which was written by human beings who made use of AI-generated summaries — was still in draft form.
The most recent draft was presented symbolically at the end of the closing Mass to the youngest participant, Joshua Eka Pramudya, a 25-year-old from Indonesia.
After the writing team makes all the changes proposed at the assembly, the text will be reviewed by members of the Federation of Asian Bishops’ Conferences (FABC) and sent to the Vatican, which has set a March 31 deadline for continental stage submissions.